Written By:
Gopal Erinjippurath
The Role of AI in Fireproofing Forest Carbon
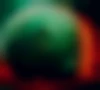
Since the Guardian article critiquing forest carbon projects and carbon credits, there has been a flurry of renewed focus on monitoring, reporting, and verification. At the moment, ensuring the ground truth of forest restoration claims and verifying additionality are top of mind for participants in the forest carbon ecosystem. We have seen significant progress across digitized tree measurements [M], forest offset reporting [R] and space derived forest carbon verification [V], what the carbon markets collectively refer to as digital MRV.
Political and business leaders are focusing too much on “restoring” rather than “protecting”, and latching on to methods they hope will offset emissions rather than prevent them. — FT, The Illusion of 1 Trillion Trees
While these technologies cover essential aspects of validity and trust in current forest carbon projects across registries, there is one aspect of forest carbon that is less talked about. It relates to forest protection from the most catastrophic form of climate induced natural hazard, wildfires. Exposure to wildfires is one of the dominant threats to the durability of forest carbon projects and the permanence of the associated offsetting credits.
Current methodologies for carbon offset durability, reversal assessment and reporting, like the AFOLU Non-Permanence Risk Tool from Verra, assume a world in which the pace of climate change is static when it comes to the risk of wildfires or extreme weather events.
However, through recent analysis, we observe the impacts of wildfire on forest carbon projects have been significant in the past decade and will grow even worse in the decades to come. Below is a quick summary of what we have learned during our research so far.
Tl;dr
❇️ Wildfire can jeopardize the quality and credibility of forest carbon projects if not quantified appropriately.
❇️❇️ Climate change and emergent 21st century bioclimatic conditions will increase wildfire risks to regions actively being considered for forest carbon projects.
❇️❇️❇️ Current wildfire modeling paradigms are limited in predictive power over decadal time horizons. Wildfire projections from interpretable AI-powered models showcase the vital capability to inform live projects and newly funded carbon projects on project non-permanence and offset durability.
Global forest carbon projects are wildfire prone
Figure 1: 190 forest carbon and agroforestry projects covering REDD+, IFM and ARR denominations studied by the carbon initiative at Sust Global, from Widespread increases in future wildfire risk to global forest carbon offset projects revealed by explainable AI, ICLR2023
Forest carbon projects are often located in areas with significant regeneration potential based on environmental conditions. When fuels are abundant and dry, fires burn more intensely and spread more quickly. The combination of fuel availability (the sheer amount of available organic matter for a wildfire to burn) and fuel aridity (how dry the fuel is) create the perfect conditions for aggressive wildfires.
Areas burned in wildfires increased particularly sharply in the western United States, where the annual average area burned in large forest fires grew by about 1,200 percent between the 1970s and the 2000s. -RFF, Wildfires in the US 101
When on fire, forest carbon project offsets are dramatically reversed
Figure 2: Cumulative sum of fire detections across the US Pacific northwest (CA, OR, WA) from Oct 2020. Source: NASA Terra and Aqua satellite data based on detections with greater than 95% confidence levels.
In 2020, wildfires in California alone emitted an estimated 112 million metric tons of carbon dioxide — roughly the same annual emissions as 24.2 million cars. -RFF, Wildfires in the US 101
Forest fires in areas where forest carbon offset projects are currently under development can create conditions for new net carbon emissions rather than carbon sequestration. Prominent organizations and standards within the voluntary carbon market, such as Verra and its Verified Carbon Standard (VCS), are well aware of this situation and have adopted the concept of buffer pools as a risk mitigation mechanism. These organizations establish guidelines and requirements for buffer pools in projects that fall under their respective standards and registries.
A buffer pool for nature-based carbon markets is a mechanism designed to mitigate the risk of carbon credit reversals in nature-based projects. Buffer pools work by requiring project developers to set aside a reserve of verified carbon credits that can be used to compensate for any future losses or reductions in sequestered CO2e emissions that may occur due to unexpected events, such as natural disasters or human activities.
The buffer pool acts as a safety net for buyers of carbon credits, ensuring that the carbon credits they purchase represent the promised volume of emissions removed from the atmosphere even if the project experiences a setback. It also provides a financial incentive for project developers to maintain and enhance the long-term carbon storage potential of their project sites, as any reductions in carbon sequestration must be compensated from the buffer pool.
We document how wildfires have depleted nearly one-fifth of the total buffer pool in less than a decade, equivalent to at least 95 percent of the program-wide contribution intended to manage all fire risks for 100 years. — Carbon Plan, California forest carbon buffer pool update
Recent research has reported the offset buffer pool for California’s forest-based offsets to be severely undercapitalized. These alarming findings inspired our climate modeling team at Sust Global to take a closer look.
Satellite data enables wildfire observations
Figure 3: Carbon projects (red) and exposure to active wildfires (orange) [Left] Carbon project boundaries with satellite observed fires from the MODIS MOD64AI L3 product [Right] Spatially filtered fires that are on non agricultural land (wildfires)
To understand wildfire impact to forest carbon at a global scale, we need to explore datasets that quantify fire observations. Satellite-derived data like the MODIS MOD64AI enables global observations of fire (more on a previous post here). However, to capture observed fire exposure, we need to filter out human-initiated agriculture burning to capture climate-induced observed wildfires.
When studying existing forest carbon projects, we observed wildfire at 67 of 190 global offset projects during the 2010 to 2021 validation period, suggesting these forest carbon projects already are exposed to significant wildfire risk. Of those, 39 projects saw more than 10% of the total project area burned, representing a substantial impact to the carbon sequestration potential of these forest carbon projects. When extending the historic analysis window to 2001 to 2021, the number of projects with more than 10% total area burned increases to 57.
Interpretable AI-powered wildfire modeling is the new frontier
Wildfires have become a common occurrence in many parts of the world, and their frequency and intensity have been on the rise in recent years. This increase in wildfire risk can be attributed to a variety of factors, but climate change is undoubtedly one of the most significant. As temperatures continue to rise, droughts become more severe, and extreme weather events become more frequent, the challenge of forecasting wildfire risk becomes even more difficult.
One approach to probabilistic estimates of wildfire exposure is through defining a monotonic relationship between an index for daily fire-prone weather (like KBDI, FWI or VPD) and wildfire probability.
We model such complex dynamics by taking millions of satellite observations of historic fires with high-resolution data on dozens of variables that contribute to fire risk — including daily precipitation and temperature, local topography and land cover, as well as fire suppress-ability and ignition sources. This is the typical AI approach — take tons of big data, fire up some GPUs, and let the model learn complex relationships that would be hard for a human to decipher.
We define our model to present two simple, interpretable layers that disaggregate overall fire risk at a location into baseline wildfire risk (Beta0) and weather-dependent wildfire risk (Beta1). This allows for a more explicit representation of the climatic impacts to wildfire exposure and allows us to model the increasing wildfire risk profile across multiple possible climate scenarios. For those interested in learning more, explore the paper here.
Figure 4: The wildfire model neural network takes as input 55 geospatial weather, bioclimatic zones, land cover, topographic characteristics, and population features to predict wildfire probability. The network combines dense layers with a final con- strained, explainable fire weather (KBDI) layer. The penultimate layer nodes serve as interpretable intercept and scaling terms for the KBDI input. From Predicting Wildfire Risk Under Novel 21st-Century Climate Conditions, AAAI2022
Through this AI based model, we can then run inference across the Earth’s landmass using bioclimatic projections for fundamental variables like temperature, precipitation and other geospatial parameters resulting a global wildfire risk exposure maps across different climate scenarios.
Figure 5: Projected mean annual wildfire risk exposure over 2023–2052 expressed as a probability under the IPCC SSP5-RCP8.5 “Business as Usual” scenario using the AI powered modeling approach. Image Credit: Sust Global, All Rights Reserved.
AI-based modeling outperforms leading climate models on the specific task of wildfire predictions
Figure 6: Validating the wildfire model (pink) on the global projects during the held out validation period from 2010–2021. We compare Area under the Curve (AUC) scores. A perfect wildfire detector would have an AUC of 1.0. We compare Sust Global’s AI model (pink) against NCAR’s CESM2 (dark grey) and CNRM’s ESM2 (light grey). CNRM-ESM2 has an AUC of 0.65, NCAR-CESM2 has an AUC of 0.68 and the Sust Global AI model has an AUC of 0.75. The wildfire model outperforms the benchmark CESM2 model. From Widespread increases in future wildfire risk to global forest carbon offset projects revealed by explainable AI, ICLR2023
We evaluate predictions on the 10 million global hold-out sample points with sample years corresponding to 2011 to 2021 (our validation window). We find that the AI model outperforms benchmark models. This improvement in performance demonstrates the model’s credibility in predicting global wildfire occurrence.
As we are interested in the model’s predictive skill in particular regions, namely the carbon offset projects, we evaluate its local performance in these regions before making any projections of future carbon project risk.
To further support application of the model to the carbon offset project collection, we benchmark predicted wildfire probabilities against projections from leading fire simulation models from CMIP6, the National Center for Atmospheric Research’s (NCAR) Community Earth System Model 2 (CESM2) model and the Center for National Meteorological Research’s Earth System Model 2 (ESM2). CESM2 and CNRM are state-of- the-art global climate models that includes a fire model estimating burned area, and we use the ensemble mean burned area from 8 runs of the climate model averaged over the validation period 2011 to 2021.
We compare Area under the Curve (AUC) scores for the interpretable AI model against NCAR’s CESM2 and CNRM’s ESM2 models. CNRM-ESM2 has an AUC of 0.65, NCAR-CESM2 has an AUC of 0.68 and the interpretable AI model has an AUC of 0.75. The wildfire model outperforms the benchmark CESM2 model. — Widespread increases in future wildfire risk to global forest carbon offset projects revealed by explainable AI, ICLR2023
Let’s take a closer look at what this projection map tells us about the near future of a forest carbon project. Below is an animation of decadal fire projections across VCS Project 868, a REDD AFOLU project covering 724K hectares of forested land in Madre de Dios, Peru. The crediting period for this project covers 2010–2040, during which there is a projected increase in risk exposure. This poses a threat to the durability of the offsets associated with this project.
Figure 7: Animation of projected annualized wildfire likelihood averaged over decades within the perimeter of a REDD forest carbon project Verra VCS 868
These AI-based innovations are available to your forest carbon projects today!
Wildfire projections at high spatial resolution enable a host of new possibilities for forest carbon projects. Mitigation measures applied to projects that account for increased wildfire exposure can lead to verifiable credits. Such methods have been applied by the Climate Action Reserve for forest carbon projects in Western US using the reduced emissions from megafires (REM) methodology. Such methodologies can be rolled out globally, and using AI-powered wildfire projections as I’ve described here can be applied to forest carbon projects to:
🔥 Assess future wildfire risk to forest carbon projects including developing nations in the global south that lack national fire risk maps
🔥🔥 Identify and score carbon risk attributed to climate catastrophes at project initiation and on repeat assessment periods
🔥🔥🔥🔥 Plan for and implement forest protection using various forest adaptation measures
This blog covers recent research covered in the paper:
T. Ballard, M. Cooper, C. Lowrie, G. Erinjippurath, Widespread increases in future wildfire risk to global forest carbon offset projects revealed by explainable AI (2023), International Conference on Learning Representations (ICLR) workshop on Tackling Climate Change with AI, May 2023, Kigali, Rwanda
Extended paper with research appendix and validation results can also be access on Arxiv here. We are open sourcing our research dataset for this study. If you are interested in exploring the source datasets and wildfire modeling datasets, reach out to support@sustglobal.com with Forest Carbon in the title or hit me up on LinkedIn.
Big thanks to the wonderfully gifted climate data scientists Tristan Ballard, Matt Cooper and Chris Lowrie, and Jess Brookes for her support on this post and the team at Sust Global for their tireless work on this carbon initiative, for their inputs to this post and for bringing this body of research to life.