Written By:
Mike Sierks
Wind power in a warming world: Leveraging Geospatial AI for wind investment insights
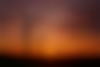
Executive Summary
Wind farms built today will face significant climate change-driven wind pattern shifts during their 30+ years in operation. Yet the industry continues to rely on historical data for siting and investment decisions—a paradox that poses increasing financial risk
Climate model output can’t be directly leveraged by the wind industry since the data is too coarse (~100km) and contains biases relative to our historical climate observations– resulting in a lack of decision-useful forward-looking wind speed data
Sust Global bridges this forward-looking gap by harnessing cutting-edge machine learning—specifically, Denoising Diffusion Probabilistic Models (DDPMs)—to transform coarse climate model simulations into high-resolution (5km) wind projections and reduce historical error by >30% compared to leading model alternatives
High-resolution and bias-corrected wind speed projections out to 2050 enable investors to integrate climate change into their diligence and financial workflows increasing individual investor performance and bolstering confidence in the broader energy transition
A case study at a European wind farm highlights the risk of relying on backwards-looking data for future investments. Relative to historical data, we find that wind speeds could decrease by 3% on average by 2040, resulting in a 10% reduction in power generation. Relying on historical data overvalues the site by >5% - posing real underperformance risk to investors.
The Problem: Yesterday's Data for Tomorrow's Wind
Wind energy is a cornerstone of global decarbonization. In Europe alone, it supplies more than 12% of electricity demand and is expected to exceed 30% by the end of this decade. Meeting ambitious climate targets requires not just more turbines, but smarter deployment across the landscape.
Yet as investment accelerates, a critical contradiction persists: wind farms being financed today—with operational lifespans of 30 years or more—are still being sited using datasets from the past despite the large wind pattern shifts expected to be brought about by climate change. Given that a turbine built today will be spinning in 2050, we ask the following questions: (1) Is historical data alone still sufficient for wind investment decision making, and if not, (2) to what extent can climate models add value?
Research has indicated that wind patterns are projected to shift in complex and regionally variable ways. Despite this, climate models are fundamentally ill-suited to provide value for wind investors for two fundamental reasons:
The resolution gap: Climate models simulate the atmosphere on grids roughly 100 kilometers wide—far too coarse to capture the local terrain features (ridgelines, channels, coastal transitions) that define wind behavior at the scale of a real-world turbine. Given that wind farms are built on scales of kilometers, higher resolution data is required to screen investments at the asset-level.
Inherent biases: Even at their native resolution, climate models contain systematic biases that must be corrected before the projections become practical.
Changing winds aren’t merely an artifact of climate change—but a source of financial uncertainty. If wind patterns shift even modestly over a project's lifetime, the impacts cascade through revenue projections, debt service coverage ratios, and investment returns. For example, a projected capacity factor decrease to 36% from 39% could determine whether a site clears investment thresholds or meets return hurdles under climate stress tests.
Our Solution: Diffusion Models for Wind Downscaling
Traditional downscaling techniques try to bridge climate model gaps through physically-based regional models or statistical methods. But these approaches are computationally expensive and challenging to run at a global scale.
To overcome these difficulties, we developed a novel approach by adapting recent breakthroughs in generative AI. Our method centers on a Denoising Diffusion Probabilistic Model (DDPM)—the same class of algorithms behind tools like DALL·E and Stable Diffusion—reconfigured to work with geospatial climate data.
Here, we integrate disparate geospatial data such as climate simulations, historical reanalysis, and remotely sensed terrain data to generate high-resolution (5km), bias-corrected projections of daily wind speed and power generation across Europe.
By combining multimodal Earth system data with cutting-edge ML architectures, our approach bridges the gap between coarse-resolution climate models and the fine-scale information required for robust wind energy planning.
Validation: DDPM models can resolve both large- and local scale wind patterns better than existing methods
We trained our model on historical data from 2007-2012, validated on 2013, and tested on 2001 across a domain covering most of Europe. To establish credibility, we benchmarked against two common approaches:
Bilinear interpolation—a simplistic way of downscaling coarse data
A CNN-based super-resolution network specifically designed for high-resolution wind speed projections
The results were decisive. On unseen test data, our DDPM-based approach:
Reduced mean squared error (MSE) by ~30% compared to the CNN
Improved structural similarity (SSIM) and peak signal-to-noise ratio (PSNR) by 5-6%
Significantly reduced spatial bias, particularly in complex terrain and high-wind regions
Most importantly, when analyzing bias in key wind energy regions such as the UK and Northwestern Europe, our model maintained error rates within ±10% of the high-resolution reference data. Outside these prime areas, most of the domain exhibited biases within ±20%, considerably outperforming traditional methods which showed errors exceeding 30% in many regions.
Future Insights: Wind Power in 2040 and Beyond
After validating our approach against the historical record, we applied the model to NASA-NEX-GDDP CMIP6 climate simulations under the SSP5-8.5 high emissions scenario. This generated high-resolution wind projections across Europe for the period 2035-2049.
To translate these wind fields into actionable insights for the energy sector, we employed two methods:
Turbine-specific power curve mapping: Converting wind speed into generation estimates using manufacturer-specific power curves (Vestas V112/3000, Gamesa G128/4500, etc.)
Wind power density calculations: Computing the power available per square meter of turbine swept area—a metric that accounts for the cubic relationship between wind speed and power potential
To highlight the practical use of downscaled forward-looking wind projections, we examine a simplified case study. Here, we highlight the risks of using backward looking data for investing in wind power under climate change. At a potential (anonymized) European wind farm, winds are projected to decrease relative to historical baseline by 1.5% by 2030 and 3% by 2040. This change may initially appear insignificant, however, the cubic relationship between wind speed changes and wind power generation mean that small but persistent changes in wind speed lead to substantial changes in power output.
When we examine the median annual power generation at this site over three different periods — we find generation to decrease by 5% and 10% respectively by 2030 and 2040.
And so, we see that in this situation, extrapolating historical data rather than using forward-looking projections results in overvaluing the project by ~5%. The issue here isn’t that one site may underperform, but that if wind projects are systemically performing differently than expected, it could lead to decreasing investment in the industry which we know to be critical to global decarbonization. In addition project underperformance leaving individual investors exposed to potential losses, systemic underperformance relative to expectations could lead to decreasing investment across the wind industry harming global decarbonization efforts. Conversely, targeting investment to sites where output will increase and providing transparency when underperformance risks are present will increase investor performance and bolster confidence in the energy transition.
Building a Climate Intelligence Platform
The model we've developed is just the beginning of what's possible at the intersection of climate science and machine learning. We're actively expanding this work in three directions:
Ensemble integration: Applying our approach across multiple climate models to quantify not just mean outcomes but the range of uncertainty—essential for robust risk modeling
Global coverage: Extending beyond Europe to cover North America, Asia, and critical offshore wind corridors like the North Sea and U.S. Gulf Coast
Industry validation: Partnering with infrastructure investors to refine our projections using proprietary wind farm performance data—bridging the gap between simulation and operations
Our long-term vision is to create a comprehensive, climate-adjusted layer of wind intelligence that informs decisions across the renewable energy lifecycle—from prospecting and development through financing, operations, and eventual repowering.
Conclusion: Machine Learning That Moves Markets
In recent years, generative AI has transformed fields from creative media to protein design. But its application to Earth system science—and particularly to climate resilience—is just beginning to reveal its potential.
What we're building is more than just a novel downscaling technique. It's a framework for engaging differently with climate risk: not just as a reporting scenario, but as a spatial, quantifiable, and actionable landscape of opportunity.
By fusing climate projections with high-resolution geospatial modeling, we can begin to ask—and answer—the kinds of questions that will shape the next three decades of energy infrastructure:
Where will wind resources grow or decline?
And how can we steer capital toward the places that will thrive under future conditions?
The answers aren't in the past. But with the right tools, they're already becoming visible—and actionable—today.